spring 2025: cs-5440
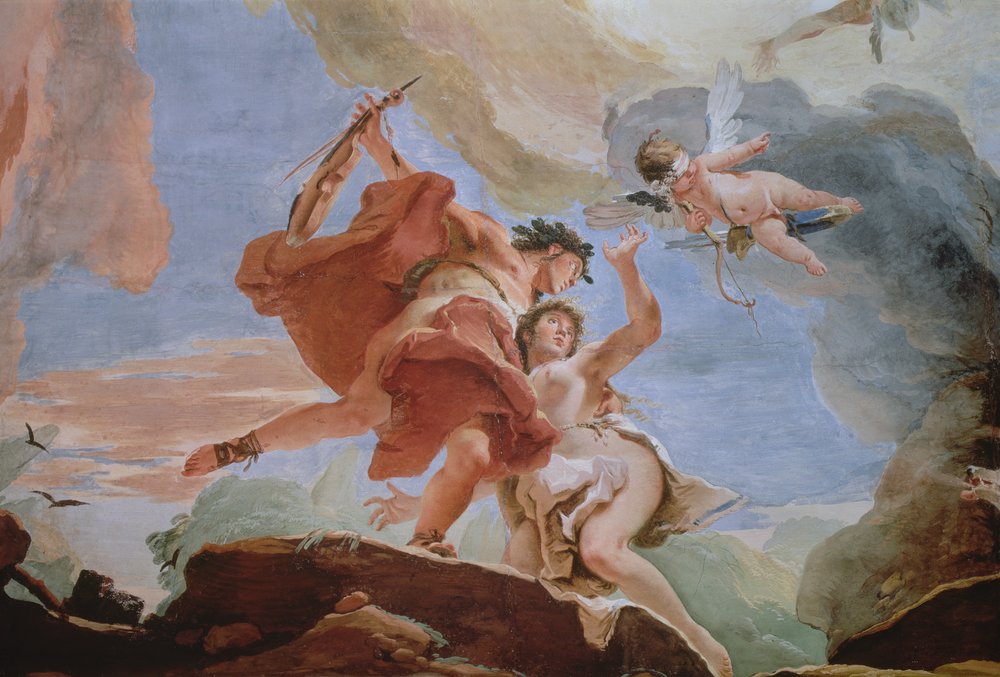
Logistics:
Instructors: | Subhashis Banerjee, Aalok Thakkar |
Credits: | 4 |
Classes: | Mondays and Wednesdays, 10:10am to 11:40pm |
Classsroom: | AC-04-LR-406 |
Teaching Assistant: | Divij Khaitan (divij.khaitan_asp25@ashoka.edu.in) |
Course Description:
In the last few years machine learning techniques have been extremely successful in applications such as image recognition and object detection, autonomous guidance, natural language understanding and translation, radiology and medical diagnosis, targeting and recommendation systems, credit scoring and risk assessment etc. They are also beginning to see applications in health analytics, econometrics and policy. As the scope of applications expand beyond computer science into data driven decisions about humans and policy advocacy, we need better understanding of the risks and potential negative fallouts. This course will be a formal enquiry into ethics of data driven decision making that affect people.
In the first part of the course we will analyse the shortcomings of the `prediction only' paradigm. We will try to formalise the notions of predictability vs reliability, and examine the possibilities of defining suitable reliability measures. In this context, we will study adversarial attacks and the possibilities of Out-Of-Distribution (OOD) samples. We will also investigate to what extent `causal inference' can enhance reliability. We will try to examine the ethical issues in building `autonomous systems' -- such as self driving cars or even weapons -- based solely on high prediction rates of modern machine learning systems (deep convolutional neural networks).
In the second part of the course we will concentrate on fairness and bias. We will try to fomalise the criteria of fairness based on the understanding of discrimination that exist in the social sciences and law. We will see that unless the data lie in narrow well-behaved manifolds, most reasonable characterisations of fairness are impossible to achieve in data driven decision making. We will also study the various types of biases that can creep into algorithmic decision making - including data bias, algorithmic focus bias, processing bias, transfer context bias and emergent bias.
We will review the spate of algorithmic interventions that have been proposed to ensure fairness and reduce bias and discuss the limitations of these approaches. We will have open discussions on how then to promote fairness and contain bias risks in data-driven decision making, and discuss the related notions of privacy, accountability, interpretabilty and transparency. We will also discuss the obvious question that follows - alternatively, is it possible to achieve fairness by being data blind? Finally, we will focus on the role of measurements in mitigating both reliability and fairness risks.
Grading Rubric: The course will have reading, discussions and quizzes for the entire semester. In addition, each participant will be required to scribe all topics and do a term-paper. Evaluations will be based on peer and instructor review of class participation, quality of reports and presentations.
- Quizzes: 20%
- Scribing and class-participation: 40%
- Term paper: 40%
Attendance Policy: The course will require 100% attendance. Class participation will count towards grading. There will be no make-up provisions for missed quizzes and class participation for whatever reason.